End of EU-funded COST Action CA18131 (ML4Microbiome)
August 21, 2023
2023 · research, networking, collaboration, microbiome, machine learning · projects
After multiple years of successful and stimulating collaboration, our EU COST Action on “Statistical and Machine Learning Techniques for Human Microbiome Studies” has come to an end.
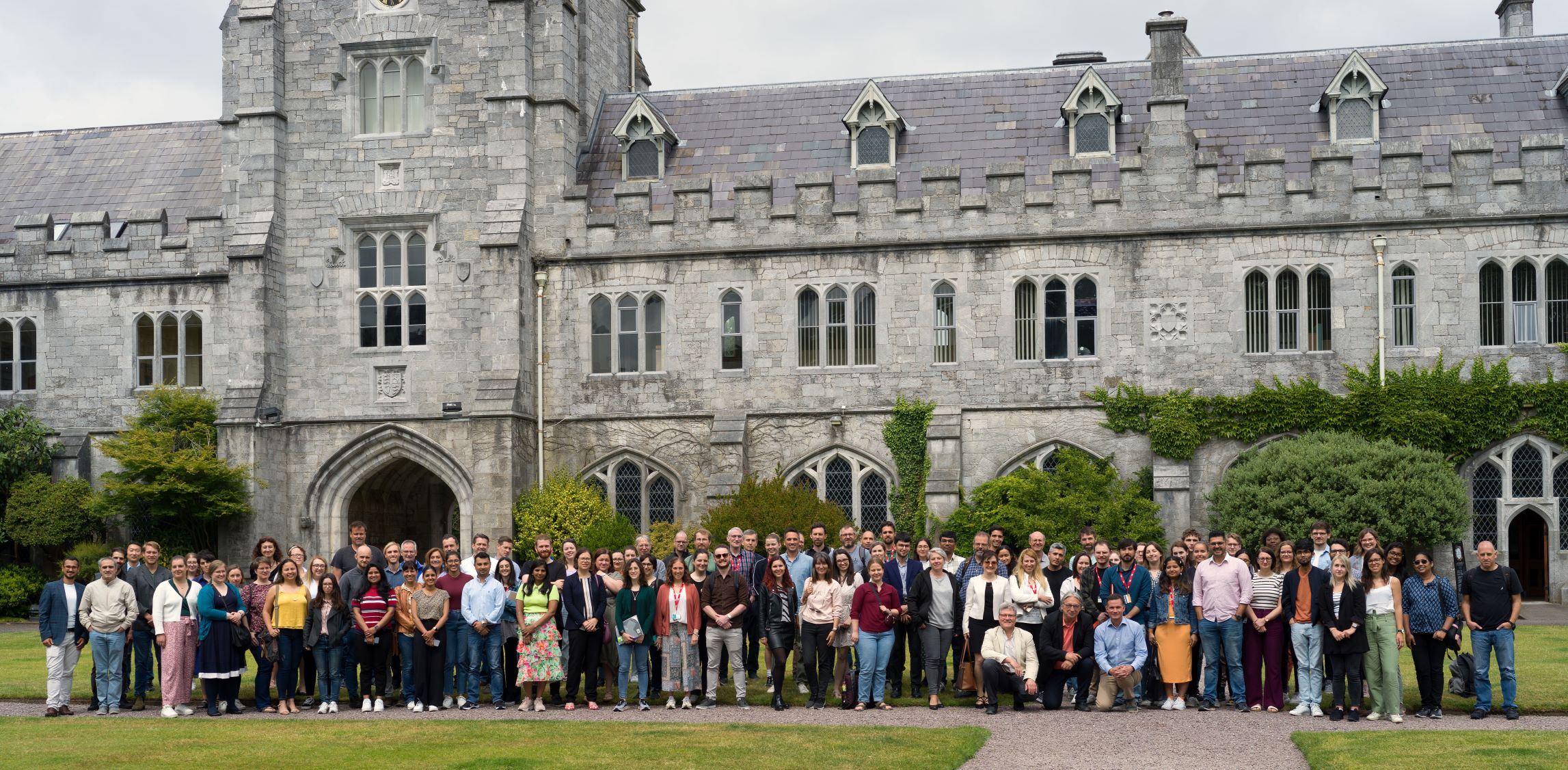
I joined the network in the final stage of my PhD and had no prior experience with COST actions, however, my involvement in this network proved to be very enriching. Engaging in various activities such as working groups and seminars broadened my academic perspective and improved my skills in project management and interdisciplinary communication. Through networking, I established valuable collaborations, contributed to publications, and presented at international events. Reflecting on this experience, I appreciate the opportunities it provided for collaboration and interdisciplinary exchange as I transition into the next stage of my academic career.
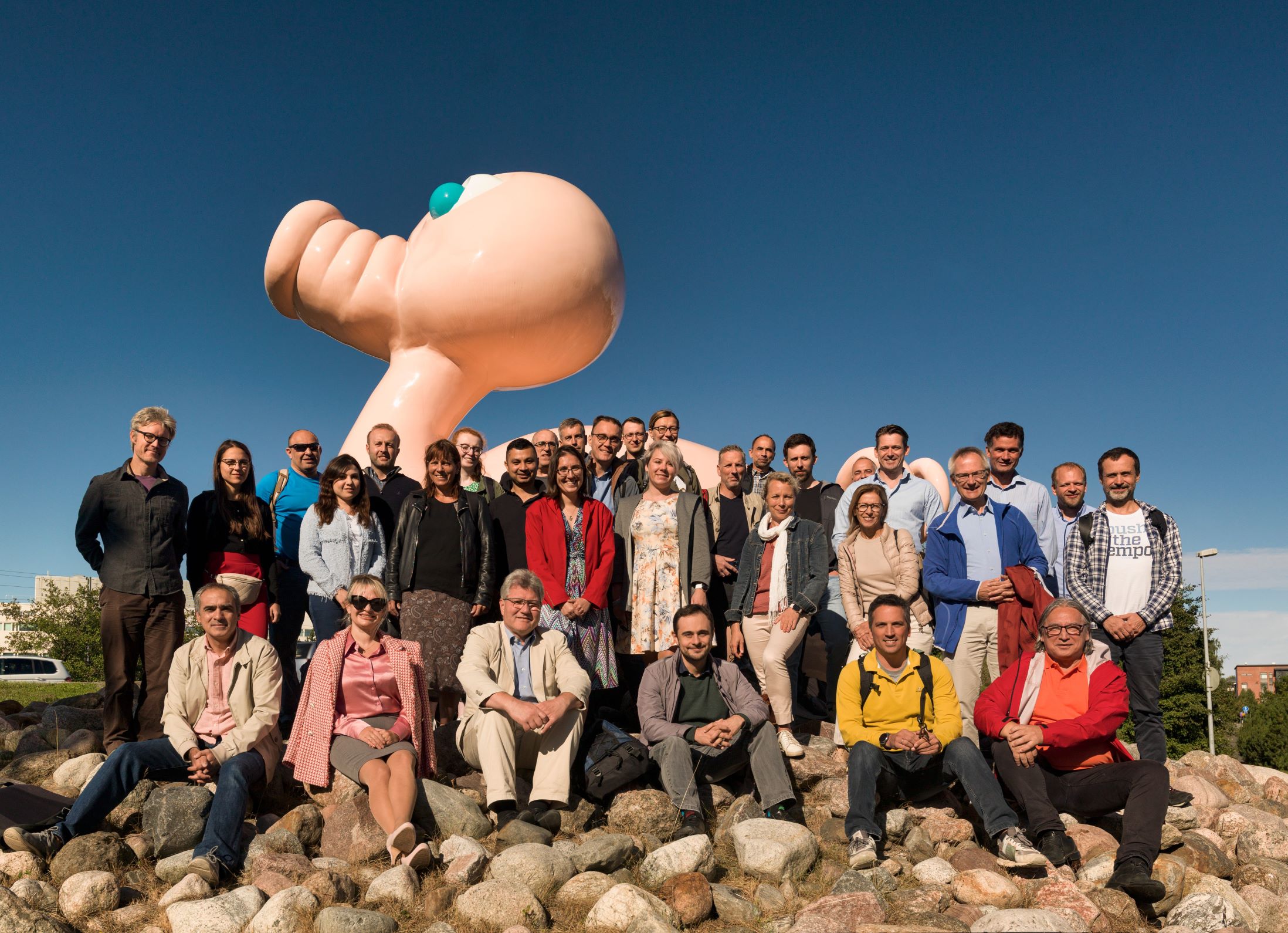
Link to COST Action project: https://cost.eu/actions/CA18131/
Official website: https://ml4microbiome.eu/
References
2023
- Machine learning approaches in microbiome research: challenges and best practicesGeorgios Papoutsoglou, Sonia Tarazona, Marta B. Lopes, Thomas Klammsteiner, Eliana Ibrahimi, Julia Eckenberger, Pierfrancesco Novielli, Alberto Tonda, Andrea Simeon, Rajesh Shigdel, Stéphane Béreux, Giacomo Vitali, Sabina Tangaro, Leo Lahti, Andriy Temko, Marcus J. Claesson, and Magali BerlandFrontiers in Microbiology, 2023
Microbiome data predictive analysis within a machine learning (ML) workflow presents numerous domain-specific challenges involving preprocessing, feature selection, predictive modeling, performance estimation, model interpretation, and the extraction of biological information from the results. To assist decision-making, we offer a set of recommendations on algorithm selection, pipeline creation and evaluation, stemming from the COST Action ML4Microbiome. We compared the suggested approaches on a multi-cohort shotgun metagenomics dataset of colorectal cancer patients, focusing on their performance in disease diagnosis and biomarker discovery. It is demonstrated that the use of compositional transformations and filtering methods as part of data preprocessing does not always improve the predictive performance of a model. In contrast, the multivariate feature selection, such as the Statistically Equivalent Signatures algorithm, was effective in reducing the classification error. When validated on a separate test dataset, this algorithm in combination with random forest modeling, provided the most accurate performance estimates. Lastly, we showed how linear modeling by logistic regression coupled with visualization techniques such as Individual Conditional Expectation (ICE) plots can yield interpretable results and offer biological insights. These findings are significant for clinicians and non-experts alike in translational applications.
@article{papoutsoglou_machine_2023, title = {Machine learning approaches in microbiome research: challenges and best practices}, volume = {14}, copyright = {All rights reserved}, issn = {1664-302X}, shorttitle = {Machine learning approaches in microbiome research}, url = {https://www.frontiersin.org/articles/10.3389/fmicb.2023.1261889}, doi = {10.3389/fmicb.2023.1261889}, urldate = {2023-09-22}, journal = {Frontiers in Microbiology}, author = {Papoutsoglou, Georgios and Tarazona, Sonia and Lopes, Marta B. and Klammsteiner, Thomas and Ibrahimi, Eliana and Eckenberger, Julia and Novielli, Pierfrancesco and Tonda, Alberto and Simeon, Andrea and Shigdel, Rajesh and Béreux, Stéphane and Vitali, Giacomo and Tangaro, Sabina and Lahti, Leo and Temko, Andriy and Claesson, Marcus J. and Berland, Magali}, year = {2023}, publisher = {Frontiers}, dimensions = true, }
- Advancing microbiome research with machine learning: key findings from the ML4Microbiome COST actionDomenica D’Elia, Jaak Truu, Leo Lahti, Magali Berland, Georgios Papoutsoglou, Michelangelo Ceci, Aldert Zomer, Marta B. Lopes, Eliana Ibrahimi, Aleksandra Gruca, Alina Nechyporenko, Marcus Frohme, Thomas Klammsteiner, Enrique Carrillo-de Santa Pau, Laura Judith Marcos-Zambrano, Karel Hron, Gianvito Pio, Andrea Simeon, Ramona Suharoschi, Isabel Moreno-Indias, Andriy Temko, Miroslava Nedyalkova, Elena-Simona Apostol, Ciprian-Octavian Truică, Rajesh Shigdel, Jasminka Hasić Telalović, Erik Bongcam-Rudloff, Piotr Przymus, Naida Babić Jordamović, Laurent Falquet, Sonia Tarazona, Alexia Sampri, Gaetano Isola, David Pérez-Serrano, Vladimir Trajkovik, Lubos Klucar, Tatjana Loncar-Turukalo, Aki S. Havulinna, Christian Jansen, Randi J. Bertelsen, and Marcus Joakim ClaessonFrontiers in Microbiology, 2023
The rapid development of machine learning (ML) techniques has opened up the data-dense field of microbiome research for novel therapeutic, diagnostic, and prognostic applications targeting a wide range of disorders, which could substantially improve healthcare practices in the era of precision medicine. However, several challenges must be addressed to exploit the benefits of ML in this field fully. In particular, there is a need to establish “gold standard” protocols for conducting ML analysis experiments and improve interactions between microbiome researchers and ML experts. The Machine Learning Techniques in Human Microbiome Studies (ML4Microbiome) COST Action CA18131 is a European network established in 2019 to promote collaboration between discovery-oriented microbiome researchers and data-driven ML experts to optimize and standardize ML approaches for microbiome analysis. This perspective paper presents the key achievements of ML4Microbiome, which include identifying predictive and discriminatory ‘omics’ features, improving repeatability and comparability, developing automation procedures, and defining priority areas for the novel development of ML methods targeting the microbiome. The insights gained from ML4Microbiome will help to maximize the potential of ML in microbiome research and pave the way for new and improved healthcare practices.
@article{delia_advancing_2023, title = {Advancing microbiome research with machine learning: key findings from the ML4Microbiome COST action}, volume = {14}, copyright = {All rights reserved}, issn = {1664-302X}, shorttitle = {Advancing microbiome research with machine learning}, url = {https://www.frontiersin.org/articles/10.3389/fmicb.2023.1257002}, doi = {10.3389/fmicb.2023.1257002}, urldate = {2023-09-21}, journal = {Frontiers in Microbiology}, author = {D’Elia, Domenica and Truu, Jaak and Lahti, Leo and Berland, Magali and Papoutsoglou, Georgios and Ceci, Michelangelo and Zomer, Aldert and Lopes, Marta B. and Ibrahimi, Eliana and Gruca, Aleksandra and Nechyporenko, Alina and Frohme, Marcus and Klammsteiner, Thomas and Pau, Enrique Carrillo-de Santa and Marcos-Zambrano, Laura Judith and Hron, Karel and Pio, Gianvito and Simeon, Andrea and Suharoschi, Ramona and Moreno-Indias, Isabel and Temko, Andriy and Nedyalkova, Miroslava and Apostol, Elena-Simona and Truică, Ciprian-Octavian and Shigdel, Rajesh and Telalović, Jasminka Hasić and Bongcam-Rudloff, Erik and Przymus, Piotr and Jordamović, Naida Babić and Falquet, Laurent and Tarazona, Sonia and Sampri, Alexia and Isola, Gaetano and Pérez-Serrano, David and Trajkovik, Vladimir and Klucar, Lubos and Loncar-Turukalo, Tatjana and Havulinna, Aki S. and Jansen, Christian and Bertelsen, Randi J. and Claesson, Marcus Joakim}, year = {2023}, publisher = {Frontiers}, dimensions = true, }
- A toolbox of machine learning software to support microbiome analysisLaura Judith Marcos Zambrano, Víctor Manuel López Molina, Burcu Bakir-Gungor*, Marcus Frohme*, Kanita Karaduzovic-Hadziabdic*, Thomas Klammsteiner*, Eliana Ibrahimi*, Leo Lahti*, Tatjana Loncar-Turukalo*, Xhilda Dhamo*, Andrea Simeon*, Alina Nechyporenko*, Gianvito Pio*, Piotr Przymus*, Alexia Sampri*, Vladimir Tihomir Trajkovik*, Oliver Aasmets, Ricardo Araujo, Ioannis Anagnostopoulos, Onder Aydemir, Magali Berland, María de la Luz Calle, Michelangelo Ceci, Hatice Duman, Aycan Gundogdu, Aki S. Havulinna, Kardokh Hama Najib Kaka Bra, Eglantina Kalluci, Sercan Karav, Daniel Lode, Marta B. Lopes, Patrick May, Bram Nap, Miroslava Nedyalkova, Inês Paciência, Lejla Pasic, Meritxell Pujolassos, Rajesh Shigdel, Antonio Susin, Ines Thiele, Ciprian-Octavian Truică, Paul Wilmes, Ercüment Yılmaz, Malik Yousef, Marcus Joakim Claesson, Jaak Truu, and Enrique Carrillo De Santa PauFrontiers in Microbiology, Nov 2023
The human microbiome has become an area of intense research due to its potential impact on human health. However, the analysis and interpretation of this data have proven to be challenging due to its complexity and high dimensionality. Machine learning (ML) algorithms can process vast amounts of data to uncover informative patterns and relationships within the data, even with limited prior knowledge. Therefore, there has been a rapid growth in the development of software specifically designed for the analysis and interpretation of microbiome data using ML techniques. These software incorporate a wide range of ML algorithms for clustering, classification, regression, or feature selection, to identify microbial patterns and relationships within the data and generate predictive models. This rapid development with a constant need for new developments and integration of new features require efforts into compile, catalog and classify these tools to create infrastructures and services with easy, transparent, and trustable standards. Here we review the state-of-the-art for ML tools applied in human microbiome studies, performed as part of the COST Action ML4Microbiome activities. This scoping review focuses on ML based software and framework resources currently available for the analysis of microbiome data in humans. The aim is to support microbiologists and biomedical scientists to go deeper into specialized resources that integrate ML techniques and facilitate future benchmarking to create standards for the analysis of microbiome data. The software resources are organized based on the type of analysis they were developed for and the ML techniques they implement. A description of each software with examples of usage is provided including comments about pitfalls and lacks in the usage of software based on ML methods in relation to microbiome data that need to be considered by developers and users. This review represents an extensive compilation to date, offering valuable insights and guidance for researchers interested in leveraging ML approaches for microbiome analysis.
@article{marcos_zambrano_toolbox_2023, title = {A toolbox of machine learning software to support microbiome analysis}, volume = {14}, copyright = {All rights reserved}, issn = {1664-302X}, url = {https://www.frontiersin.org/articles/10.3389/fmicb.2023.1250806}, doi = {10.3389/fmicb.2023.1250806}, language = {English}, urldate = {2023-11-12}, journal = {Frontiers in Microbiology}, author = {Marcos Zambrano, Laura Judith and López Molina, Víctor Manuel and Bakir-Gungor*, Burcu and Frohme*, Marcus and Karaduzovic-Hadziabdic*, Kanita and Klammsteiner*, Thomas and Ibrahimi*, Eliana and Lahti*, Leo and Loncar-Turukalo*, Tatjana and Dhamo*, Xhilda and Simeon*, Andrea and Nechyporenko*, Alina and Pio*, Gianvito and Przymus*, Piotr and Sampri*, Alexia and Trajkovik*, Vladimir Tihomir and Aasmets, Oliver and Araujo, Ricardo and Anagnostopoulos, Ioannis and Aydemir, Onder and Berland, Magali and Calle, María de la Luz and Ceci, Michelangelo and Duman, Hatice and Gundogdu, Aycan and Havulinna, Aki S. and Kaka Bra, Kardokh Hama Najib and Kalluci, Eglantina and Karav, Sercan and Lode, Daniel and Lopes, Marta B. and May, Patrick and Nap, Bram and Nedyalkova, Miroslava and Paciência, Inês and Pasic, Lejla and Pujolassos, Meritxell and Shigdel, Rajesh and Susin, Antonio and Thiele, Ines and Truică, Ciprian-Octavian and Wilmes, Paul and Yılmaz, Ercüment and Yousef, Malik and Claesson, Marcus Joakim and Truu, Jaak and Carrillo De Santa Pau, Enrique}, month = nov, year = {2023}, publisher = {Frontiers}, pages = {1250806}, dimensions = true, }
2021
- Applications of machine learning in human microbiome studies: a review on feature selection, biomarker identification, disease prediction and treatmentLaura Judith Marcos Zambrano, Kanita Karaduzovic-Hadziabdic, Tatjana Loncar-Turukalo, Piotr Przymus, Vladimir Trajkovik, Oliver Aasmets, Magali Berland, Aleksandra Gruca, Jasminka Hasic Telalovic, Hron Karel, Thomas Klammsteiner, Mikhail Kolev, Leo Lahti, Mart B. Lopes, Victor Moreno, Irina Naskinova, Elin Org, Inês Paciência, Georgios Papoutsoglou, Rajesh Shigdel, Blaz Stres, Baiba Vilne, Malik Yousef, Eftim Zdravevski, Ioannis Tsamardinos, Enrique Carrillo Santa Pau, Marcus Claesson, Isabel Moreno Indias, and Jaak TruuFrontiers in Microbiology, Feb 2021
The number of microbiome-related studies has notably increased the availability of data on human microbiome composition and function. These studies provide the essential material to deeply explore host-microbiome associations and their relation to the development and progression of various complex diseases. Improved data-analytical tools are needed to exploit all information from these biological datasets, taking into account the peculiarities of microbiome data, i.e. compositional, heterogeneous and sparse nature of these datasets. The possibility of predicting host-phenotypes based on taxonomy-informed feature selection to establish an association between microbiome and predict disease states is beneficial for personalized medicine. In this regard, machine learning (ML) provides new insights into the development of models that can be used to predict outputs, such as classification and prediction in microbiology, infer host phenotypes to predict diseases and use microbial communities to stratify patients by their characterization of state-specific microbial signatures. Here we review the state-of-the-art ML methods and respective software applied in human microbiome studies, performed as part of the COST Action ML4Microbiome activities. This scoping review focuses on the application of ML in microbiome studies related to association and clinical use for diagnostics, prognostics, and therapeutics. Although the data presented here is more related to the bacterial community, many algorithms could be applied in general, regardless of the feature type. This literature and software review covering this broad topic is aligned with the scoping review methodology. The manual identification of data sources has been complemented with: (1) automated publication search through digital libraries of the three major publishers using natural language processing (NLP) Toolkit, and (2) an automated identification of relevant software repositories on GitHub and ranking of the related research papers relying on learning to rank approach.
@article{zambrano_applications_2021, title = {Applications of machine learning in human microbiome studies: a review on feature selection, biomarker identification, disease prediction and treatment}, volume = {12}, copyright = {All rights reserved}, issn = {1664-302X}, shorttitle = {Applications of machine learning in human microbiome studies}, url = {https://www.frontiersin.org/articles/10.3389/fmicb.2021.634511/abstract}, doi = {10.3389/fmicb.2021.634511}, language = {English}, urldate = {2021-02-17}, journal = {Frontiers in Microbiology}, author = {Zambrano, Laura Judith Marcos and Karaduzovic-Hadziabdic, Kanita and Loncar-Turukalo, Tatjana and Przymus, Piotr and Trajkovik, Vladimir and Aasmets, Oliver and Berland, Magali and Gruca, Aleksandra and Hasic Telalovic, Jasminka and Karel, Hron and Klammsteiner, Thomas and Kolev, Mikhail and Lahti, Leo and Lopes, Mart B. and Moreno, Victor and Naskinova, Irina and Org, Elin and Paciência, Inês and Papoutsoglou, Georgios and Shigdel, Rajesh and Stres, Blaz and Vilne, Baiba and Yousef, Malik and Zdravevski, Eftim and Tsamardinos, Ioannis and de Santa Pau, Enrique Carrillo and Claesson, Marcus and Moreno Indias, Isabel and Truu, Jaak}, month = feb, year = {2021}, dimensions = {true}, publisher = {Frontiers}, keywords = {microbiome, biomarker identification, Disease Prediction, feature selection, machine learning}, pages = {634511}, }
- Statistical and machine learning techniques in human microbiome studies: contemporary challenges and solutionsIsabel Moreno-Indias, Leo Lahti, Miroslava Nedyalkova, Ilze Elbere, Gennady V. Roshchupkin, Muhamed Adilovic, Onder Aydemir, Burcu Bakir-Gungor, Enrique Carrillo-de Santa Pau, Domenica D’Elia, Magesh S. Desai, Laurent Falquet, Aycan Gundogdu, Karel Hron, Thomas Klammsteiner, Marta B. Lopes, Laura Judith Marcos Zambrano, Cláudia Marques, Michael Mason, Patrick May, Lejla Pašić, Gianvito Pio, Sándor Pongor, Vasilis J. Promponas, Piotr Przymus, Julio Sáez-Rodríguez, Alexia Sampri, Rajesh Shigdel, Blaz Stres, Ramona Suharoschi, Jaak Truu, Ciprian-Octavian Truică, Baiba Vilne, Dimitrios P. Vlachakis, Ercüment Yılmaz, Georg Zeller, Aldert Zomer, David Gómez-Cabrero, and Marcus ClaessonFrontiers in Microbiology, Feb 2021
Human microbiome has emerged as a central research topic in human biology and biomedicine. Current microbiome studies generate high-throughput omics data across different body sites, populations, and lifetime. Whereas many of the challenges in microbiome research are similar to other high-throughput studies, the quantitative analyses need to address the heterogeneity of data, specific statistical properties, and the remarkable variation in microbiome composition across individuals and body sites. This has led to a broad spectrum of statistical and machine learning challenges that range from study design, data processing, and standardization to analysis, modeling, cross-study comparison, prediction, data science ecosystems, and reproducible reporting. Nevertheless, although many statistics and machine learning approaches and tools have been developed, new techniques are needed to deal with emerging applications and the vast heterogeneity of microbiome data. We review and discuss emerging applications of statistical and machine learning techniques in human microbiome studies and introduce the COST Action CA18131 "ML4Microbiome" that brings together microbiome researchers and machine learning experts to address current challenges such as standardisation of analysis pipelines for reproducibility of data analysis results, benchmarking, improvement, or development of existing and new tools and ontologies.
@article{moreno-indias_statistical_2021, title = {Statistical and machine learning techniques in human microbiome studies: contemporary challenges and solutions}, volume = {12}, copyright = {All rights reserved}, issn = {1664-302X}, shorttitle = {Statistical and machine learning techniques in human microbiome studies}, url = {https://www.frontiersin.org/articles/10.3389/fmicb.2021.635781/abstract}, doi = {10.3389/fmicb.2021.635781}, language = {English}, urldate = {2021-02-18}, journal = {Frontiers in Microbiology}, author = {Moreno-Indias, Isabel and Lahti, Leo and Nedyalkova, Miroslava and Elbere, Ilze and Roshchupkin, Gennady V. and Adilovic, Muhamed and Aydemir, Onder and Bakir-Gungor, Burcu and Carrillo-de Santa Pau, Enrique and D'Elia, Domenica and Desai, Magesh S. and Falquet, Laurent and Gundogdu, Aycan and Hron, Karel and Klammsteiner, Thomas and Lopes, Marta B. and Marcos Zambrano, Laura Judith and Marques, Cláudia and Mason, Michael and May, Patrick and Pašić, Lejla and Pio, Gianvito and Pongor, Sándor and Promponas, Vasilis J. and Przymus, Piotr and Sáez-Rodríguez, Julio and Sampri, Alexia and Shigdel, Rajesh and Stres, Blaz and Suharoschi, Ramona and Truu, Jaak and Truică, Ciprian-Octavian and Vilne, Baiba and Vlachakis, Dimitrios P. and Yılmaz, Ercüment and Zeller, Georg and Zomer, Aldert and Gómez-Cabrero, David and Claesson, Marcus}, month = feb, year = {2021}, publisher = {Frontiers}, keywords = {microbiome, biomarker identification, machine learning, ML4microbiome, personalized medicine}, pages = {635781}, dimensions = true, }