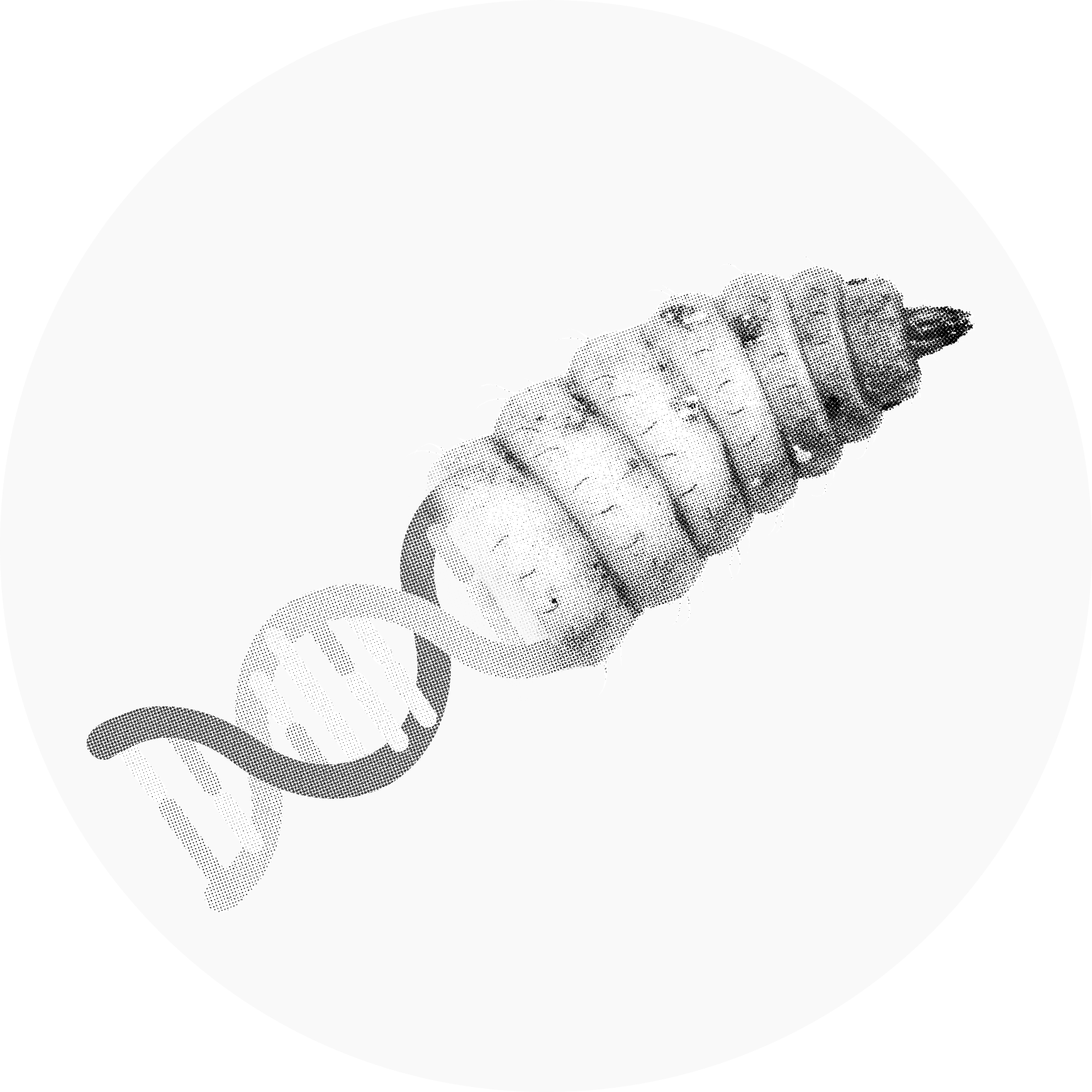
Thomas Klammsteiner
microbes | environment | data
Postdoctoral researcher
Department of Ecology
University of Innsbruck, Innsbruck, Austria
I’m a microbiologist passionate about microbial ecology and all kinds of microbe-host interactions. My current research focuses on using ‘omics methods to explore microbiota associated with industrially farmed insects, as well as understanding physicochemical dynamics in insect mass-rearing. My interests span across microbiome research, data science, and visualization, as well as driving sustainable efficiency improvements in various processes.
news
Jun 11, 2024 | New publication: Suraporn et al. (2024) Agriculture 14(6), 924 |
---|---|
Mar 21, 2024 | New publication: Praeg and Klammsteiner (2024) J Environ Manage 356, 120622 |
Feb 15, 2024 | EuroBioc2024 (European Bioconductor Conference) sticker contest winner |
Jan 1, 2024 | New publication: Gómez-Brandón et al. (2024) Waste Manage 173, 131-140 |
Dec 15, 2023 | Update for website on BSF-related topics at www.fromwastetofeed.com |
latest posts
selected publications
- The core gut microbiome of black soldier fly (Hermetia illucens) larvae raised on low-bioburden dietsThomas Klammsteiner, Andreas Walter, Tajda Bogataj, Carina D. Heussler, Blaž Stres, Florian M. Steiner, Birgit C. Schlick-Steiner, Wolfgang Arthofer, and Heribert InsamFrontiers in Microbiology, May 2020
An organism’s gut microbiome handles most of the metabolic processes associated with food intake and digestion but can also strongly affect health and behavior. A stable microbial core community in the gut provides general metabolic competences for substrate degradation and is robust against extrinsic disturbances like changing diets or pathogens. Black Soldier Fly larvae (BSFL; Hermetia illucens) are well known for their ability to efficiently degrade a wide spectrum of organic materials. The ingested substrates build up the high fat and protein content in their bodies that make the larvae interesting for the animal feedstuff industry. In this study, we subjected BSFL to three distinct types of diets carrying a low bioburden and assessed the diets’ impact on larval development and on the composition of the bacterial and archaeal gut community. No significant impact on the gut microbiome across treatments pointed us to the presence of a predominant core community backed by a diverse spectrum of low-abundance taxa. Actinomyces spp., Dysgonomonas spp., and Enterococcus spp. as main members of this community provide various functional and metabolic skills that could be crucial for the thriving of BSFL in various environments. This indicates that the type of diet could play a lesser role in guts of BSFL than previously assumed and that instead a stable autochthonous collection of bacteria provides the tools for degrading of a broad range of substrates. Characterizing the interplay between the core gut microbiome and BSFL helps to understand the involved degradation processes and could contribute to further improving large-scale BSFL rearing.
@article{klammsteiner_core_2020, title = {The core gut microbiome of black soldier fly (<i>Hermetia illucens</i>) larvae raised on low-bioburden diets}, volume = {11}, copyright = {Creative Commons Attribution-NonCommercial-NoDerivatives 4.0 International License (CC-BY-NC-ND)}, issn = {1664-302X}, url = {https://www.frontiersin.org/articles/10.3389/fmicb.2020.00993/full?&utm_source=Email_to_authors_&utm_medium=Email&utm_content=T1_11.5e1_author&utm_campaign=Email_publication&field=&journalName=Frontiers_in_Microbiology&id=499002}, doi = {10.3389/fmicb.2020.00993}, language = {English}, urldate = {2020-05-21}, journal = {Frontiers in Microbiology}, author = {Klammsteiner, Thomas and Walter, Andreas and Bogataj, Tajda and Heussler, Carina D. and Stres, Blaž and Steiner, Florian M. and Schlick-Steiner, Birgit C. and Arthofer, Wolfgang and Insam, Heribert}, month = may, year = {2020}, publisher = {Frontiers}, keywords = {16S amplicon sequencing, Animal feedstuff, Circular economy, microbial communities, Waste valorization, AGIV, Actinomyces, Larval metabolism}, pages = {993}, dimensions = true, }
- Suitability of black soldier fly frass as soil amendment and implication for organic waste hygienizationThomas Klammsteiner, Veysel Turan, Marina Fernández-Delgado Juárez, Simon Oberegger, and Heribert InsamAgronomy, Oct 2020
Because of its nutritious properties, the black soldier fly has emerged as one of the most popular species in advancing circular economy through the re-valorization of anthropogenic organic wastes to insect biomass. Black soldier fly frass accumulates as a major by-product in artificial rearing set-ups and harbors great potential to complement or replace commercial fertilizers. We applied frass from larvae raised on different diets in nitrogen-equivalent amounts as soil amendment, comparing it to NH4NO3 fertilizer as a control. While the soil properties did not reveal any difference between mineral fertilizer and frass, principal component analysis showed significant differences that are mainly attributed to nitrate and dissolved nitrogen contents. We did not find significant differences in the growth of perennial ryegrass between the treatments, indicating that frass serves as a rapidly acting fertilizer comparable to NH4NO3. While the abundance of coliform bacteria increased during frass maturation, after application to the soil, they were outcompeted by gram-negatives. We thus conclude that frass may serve as a valuable fertilizer and does not impair the hygienic properties of soils.
@article{klammsteiner_suitability_2020, title = {Suitability of black soldier fly frass as soil amendment and implication for organic waste hygienization}, volume = {10}, copyright = {http://creativecommons.org/licenses/by/3.0/}, url = {https://www.mdpi.com/2073-4395/10/10/1578}, doi = {10.3390/agronomy10101578}, language = {en}, number = {10}, urldate = {2020-10-16}, journal = {Agronomy}, author = {Klammsteiner, Thomas and Turan, Veysel and Juárez, Marina Fernández-Delgado and Oberegger, Simon and Insam, Heribert}, month = oct, year = {2020}, publisher = {MDPI}, keywords = {circular economy, organic waste, animal feedstuff, fertilizer, greenhouse, insect larva}, pages = {1578}, dimensions = true, }
- Impact of processed food (canteen and oil wastes) on the development of black soldier fly (Hermetia illucens) larvae and their gut microbiome functionsThomas Klammsteiner, Andreas Walter, Tajda Bogataj, Carina D. Heussler, Blaž Stres, Florian M. Steiner, Birgit C. Schlick-Steiner, and Heribert InsamFrontiers in Microbiology, Jan 2021
Canteens represent an essential food supply hub for educational institutions, companies, and business parks. Many people in these locations rely on a guaranteed service with consistent quality. It is an ongoing challenge to satisfy the demand for sufficient serving numbers, portion sizes, and menu variations to cover food intolerances and different palates of customers. However, overestimating this demand or fluctuating quality of dishes leads to an inevitable loss of unconsumed food due to leftovers. In this study, the food waste fraction of canteen leftovers was identified as an optimal diet for black soldier fly (Hermetia illucens) larvae based on 50% higher consumption and 15% higher waste reduction indices compared with control chicken feed diet. Although the digestibility of food waste was nearly twice as high, the conversion efficiency of ingested and digested chicken feed remains unparalleled (17.9 ± 0.6 and 37.5 ± 0.9 in CFD and 7.9 ± 0.9 and 9.6 ± 1.0 in FWD, respectively). The oil separator waste fraction, however, inhibited biomass gain by at least 85% and ultimately led to a larval mortality of up to 96%. In addition to monitoring larval development, we characterized physicochemical properties of pre- and post-process food waste substrates. High-throughput amplicon sequencing identified Firmicutes, Proteobacteria, and Bacteroidota as the most abundant phyla, and Morganella sp., Acinetobacter sp., and certain Lactobacillales species were identified as indicator species. By using metagenome imputation, we additionally gained insights into the functional spectrum of gut microbial communities. We anticipate that the results will contribute to the development of decentralized waste-management sites that make use of larvae to process food waste as it has become common practice for biogas plants.
@article{klammsteiner_impact_2021, title = {Impact of processed food (canteen and oil wastes) on the development of black soldier fly (<i>Hermetia illucens</i>) larvae and their gut microbiome functions}, volume = {12}, copyright = {All rights reserved}, issn = {1664-302X}, url = {https://www.frontiersin.org/articles/10.3389/fmicb.2021.619112/full}, doi = {10.3389/fmicb.2021.619112}, language = {English}, urldate = {2021-02-04}, journal = {Frontiers in Microbiology}, author = {Klammsteiner, Thomas and Walter, Andreas and Bogataj, Tajda and Heussler, Carina D. and Stres, Blaž and Steiner, Florian M. and Schlick-Steiner, Birgit C. and Insam, Heribert}, month = jan, year = {2021}, publisher = {Frontiers}, keywords = {16S amplicon sequencing, Animal feedstuff, Circular economy, Growth parameters, Metabolism, microbial communities, Oil waste, Waste valorization}, pages = {619112}, dimensions = true, }
- Statistical and machine learning techniques in human microbiome studies: contemporary challenges and solutionsIsabel Moreno-Indias, Leo Lahti, Miroslava Nedyalkova, Ilze Elbere, Gennady V. Roshchupkin, Muhamed Adilovic, Onder Aydemir, Burcu Bakir-Gungor, Enrique Carrillo-de Santa Pau, Domenica D’Elia, Magesh S. Desai, Laurent Falquet, Aycan Gundogdu, Karel Hron, Thomas Klammsteiner, Marta B. Lopes, Laura Judith Marcos Zambrano, Cláudia Marques, Michael Mason, Patrick May, Lejla Pašić, Gianvito Pio, Sándor Pongor, Vasilis J. Promponas, Piotr Przymus, Julio Sáez-Rodríguez, Alexia Sampri, Rajesh Shigdel, Blaz Stres, Ramona Suharoschi, Jaak Truu, Ciprian-Octavian Truică, Baiba Vilne, Dimitrios P. Vlachakis, Ercüment Yılmaz, Georg Zeller, Aldert Zomer, David Gómez-Cabrero, and Marcus ClaessonFrontiers in Microbiology, Feb 2021
Human microbiome has emerged as a central research topic in human biology and biomedicine. Current microbiome studies generate high-throughput omics data across different body sites, populations, and lifetime. Whereas many of the challenges in microbiome research are similar to other high-throughput studies, the quantitative analyses need to address the heterogeneity of data, specific statistical properties, and the remarkable variation in microbiome composition across individuals and body sites. This has led to a broad spectrum of statistical and machine learning challenges that range from study design, data processing, and standardization to analysis, modeling, cross-study comparison, prediction, data science ecosystems, and reproducible reporting. Nevertheless, although many statistics and machine learning approaches and tools have been developed, new techniques are needed to deal with emerging applications and the vast heterogeneity of microbiome data. We review and discuss emerging applications of statistical and machine learning techniques in human microbiome studies and introduce the COST Action CA18131 "ML4Microbiome" that brings together microbiome researchers and machine learning experts to address current challenges such as standardisation of analysis pipelines for reproducibility of data analysis results, benchmarking, improvement, or development of existing and new tools and ontologies.
@article{moreno-indias_statistical_2021, title = {Statistical and machine learning techniques in human microbiome studies: contemporary challenges and solutions}, volume = {12}, copyright = {All rights reserved}, issn = {1664-302X}, shorttitle = {Statistical and machine learning techniques in human microbiome studies}, url = {https://www.frontiersin.org/articles/10.3389/fmicb.2021.635781/abstract}, doi = {10.3389/fmicb.2021.635781}, language = {English}, urldate = {2021-02-18}, journal = {Frontiers in Microbiology}, author = {Moreno-Indias, Isabel and Lahti, Leo and Nedyalkova, Miroslava and Elbere, Ilze and Roshchupkin, Gennady V. and Adilovic, Muhamed and Aydemir, Onder and Bakir-Gungor, Burcu and Carrillo-de Santa Pau, Enrique and D'Elia, Domenica and Desai, Magesh S. and Falquet, Laurent and Gundogdu, Aycan and Hron, Karel and Klammsteiner, Thomas and Lopes, Marta B. and Marcos Zambrano, Laura Judith and Marques, Cláudia and Mason, Michael and May, Patrick and Pašić, Lejla and Pio, Gianvito and Pongor, Sándor and Promponas, Vasilis J. and Przymus, Piotr and Sáez-Rodríguez, Julio and Sampri, Alexia and Shigdel, Rajesh and Stres, Blaz and Suharoschi, Ramona and Truu, Jaak and Truică, Ciprian-Octavian and Vilne, Baiba and Vlachakis, Dimitrios P. and Yılmaz, Ercüment and Zeller, Georg and Zomer, Aldert and Gómez-Cabrero, David and Claesson, Marcus}, month = feb, year = {2021}, publisher = {Frontiers}, keywords = {microbiome, biomarker identification, machine learning, ML4microbiome, personalized medicine}, pages = {635781}, dimensions = true, }
- A toolbox of machine learning software to support microbiome analysisLaura Judith Marcos Zambrano, Víctor Manuel López Molina, Burcu Bakir-Gungor*, Marcus Frohme*, Kanita Karaduzovic-Hadziabdic*, Thomas Klammsteiner*, Eliana Ibrahimi*, Leo Lahti*, Tatjana Loncar-Turukalo*, Xhilda Dhamo*, Andrea Simeon*, Alina Nechyporenko*, Gianvito Pio*, Piotr Przymus*, Alexia Sampri*, Vladimir Tihomir Trajkovik*, Oliver Aasmets, Ricardo Araujo, Ioannis Anagnostopoulos, Onder Aydemir, Magali Berland, María de la Luz Calle, Michelangelo Ceci, Hatice Duman, Aycan Gundogdu, Aki S. Havulinna, Kardokh Hama Najib Kaka Bra, Eglantina Kalluci, Sercan Karav, Daniel Lode, Marta B. Lopes, Patrick May, Bram Nap, Miroslava Nedyalkova, Inês Paciência, Lejla Pasic, Meritxell Pujolassos, Rajesh Shigdel, Antonio Susin, Ines Thiele, Ciprian-Octavian Truică, Paul Wilmes, Ercüment Yılmaz, Malik Yousef, Marcus Joakim Claesson, Jaak Truu, and Enrique Carrillo De Santa PauFrontiers in Microbiology, Nov 2023
The human microbiome has become an area of intense research due to its potential impact on human health. However, the analysis and interpretation of this data have proven to be challenging due to its complexity and high dimensionality. Machine learning (ML) algorithms can process vast amounts of data to uncover informative patterns and relationships within the data, even with limited prior knowledge. Therefore, there has been a rapid growth in the development of software specifically designed for the analysis and interpretation of microbiome data using ML techniques. These software incorporate a wide range of ML algorithms for clustering, classification, regression, or feature selection, to identify microbial patterns and relationships within the data and generate predictive models. This rapid development with a constant need for new developments and integration of new features require efforts into compile, catalog and classify these tools to create infrastructures and services with easy, transparent, and trustable standards. Here we review the state-of-the-art for ML tools applied in human microbiome studies, performed as part of the COST Action ML4Microbiome activities. This scoping review focuses on ML based software and framework resources currently available for the analysis of microbiome data in humans. The aim is to support microbiologists and biomedical scientists to go deeper into specialized resources that integrate ML techniques and facilitate future benchmarking to create standards for the analysis of microbiome data. The software resources are organized based on the type of analysis they were developed for and the ML techniques they implement. A description of each software with examples of usage is provided including comments about pitfalls and lacks in the usage of software based on ML methods in relation to microbiome data that need to be considered by developers and users. This review represents an extensive compilation to date, offering valuable insights and guidance for researchers interested in leveraging ML approaches for microbiome analysis.
@article{marcos_zambrano_toolbox_2023, title = {A toolbox of machine learning software to support microbiome analysis}, volume = {14}, copyright = {All rights reserved}, issn = {1664-302X}, url = {https://www.frontiersin.org/articles/10.3389/fmicb.2023.1250806}, doi = {10.3389/fmicb.2023.1250806}, language = {English}, urldate = {2023-11-12}, journal = {Frontiers in Microbiology}, author = {Marcos Zambrano, Laura Judith and López Molina, Víctor Manuel and Bakir-Gungor*, Burcu and Frohme*, Marcus and Karaduzovic-Hadziabdic*, Kanita and Klammsteiner*, Thomas and Ibrahimi*, Eliana and Lahti*, Leo and Loncar-Turukalo*, Tatjana and Dhamo*, Xhilda and Simeon*, Andrea and Nechyporenko*, Alina and Pio*, Gianvito and Przymus*, Piotr and Sampri*, Alexia and Trajkovik*, Vladimir Tihomir and Aasmets, Oliver and Araujo, Ricardo and Anagnostopoulos, Ioannis and Aydemir, Onder and Berland, Magali and Calle, María de la Luz and Ceci, Michelangelo and Duman, Hatice and Gundogdu, Aycan and Havulinna, Aki S. and Kaka Bra, Kardokh Hama Najib and Kalluci, Eglantina and Karav, Sercan and Lode, Daniel and Lopes, Marta B. and May, Patrick and Nap, Bram and Nedyalkova, Miroslava and Paciência, Inês and Pasic, Lejla and Pujolassos, Meritxell and Shigdel, Rajesh and Susin, Antonio and Thiele, Ines and Truică, Ciprian-Octavian and Wilmes, Paul and Yılmaz, Ercüment and Yousef, Malik and Claesson, Marcus Joakim and Truu, Jaak and Carrillo De Santa Pau, Enrique}, month = nov, year = {2023}, publisher = {Frontiers}, pages = {1250806}, dimensions = true, }